Soil moisture plays a critical role in the Earth’s climate, ecosystem health, and agricultural productivity. However, measuring and interpreting soil moisture is not as straightforward as it may seem. At a recent LEMONTREE Science meeting, PI Pierre Gentine (Columbia University) explored the complexities of soil moisture measurement, the challenges of integrating different datasets, and the significance of long-term trends.
How Do We Measure Soil Moisture?
There are two primary methods for measuring soil moisture: in-situ observations and remote sensing techniques.
- In Situ Measurements: Precise but Challenging
In situ measurements involve placing sensors directly into the soil to measure dielectric properties, which are then calibrated using gravimetric methods (weighing soil samples before and after drying). While this method provides highly localized and detailed data, it comes with several challenges:
- Calibration Issues: Ensuring accurate readings requires proper calibration, which can be difficult over time.
- Environmental Disturbances: Animal activity, soil shifting, and sensor degradation can all impact readings.
- Spatial Heterogeneity: Soil texture, soil structure (roots, cracks etc) and moisture levels vary greatly over small distances, making a single measurement site potentially unrepresentative of a larger area.
- Remote Sensing: Large-Scale Consistency with Limitations
Remote sensing uses microwave measurements from satellites to estimate soil moisture. This can be done through:
- Passive Microwave Sensing: Detecting natural microwave emissions from the Earth’s surface.
- Active Microwave Sensing (Radar): Emitting and receiving microwave signals to infer soil moisture levels.
The advantage of remote sensing is that it provides global, consistent, and frequent measurements. However, the data are not direct measurements of soil moisture; instead, they rely on proxy indicators such as brightness temperature. Another challenges of using remote soil moisture data is separating the soil signal from the vegetation signal—someone’s noise is someone else’s signal! Vegetation creates opacity, making soil moisture retrieval tricky. We are now levDeraging this “noise” to gain insights into plant water content and ecosystem stress.
Merging Data for Long-Term Analysis
One of the biggest challenges in soil moisture research is integrating data from different sources over time. Since satellite sensors evolve across generations, their data need to be merged through bias correction techniques to ensure consistency.
For example, the European Space Agency’s Climate Change Initiative (ESA CCI) has compiled historical soil moisture data from multiple satellite missions. To make these datasets comparable, researchers apply bias corrections, aligning measurements from different sensors to create a continuous long-term dataset.
A critical issue here is gap filling—satellite data are not always continuous, and missing observations must be estimated. If this process is not done carefully, it can introduce significant errors and lead to misleading trends.
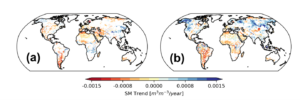
(CAN YOU ADD IN THE ESA CCI GAPFILLED LONG-TERM TRENDS GRAPHS FROM SLIDE 3)
Understanding Seasonal Trends in Soil Moisture
Annual averages of soil moisture can sometimes mask important seasonal variations. Spring and summer soil moisture trends can behave very differently, even if the overall yearly change appears minimal.
For example:
- Spring Moisture Increase: Due to earlier snowmelt caused by climate change, many regions are experiencing higher soil moisture levels in spring. This can extend the growing season with an earlier start which can influence plant productivity.
- Summer Moisture Decline: While spring moisture levels may be rising, summer can still experience depletion due to increased evaporation and plant water uptake.
- Autumn Moisture Increases: Due to rising temperatures, in some regions in late Autumn there is an increase in soil moisture as there is a shift from snow to rain with temperature. Also, the growing season is expending with temperature which can impact soil moisture levels.
This highlights the need to analyse soil moisture trends seasonally, rather than relying solely on annual averages.
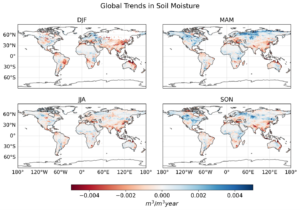
The Reliability of In Situ Measurements
While in situ measurements are often used to validate remote sensing data, they come with their own set of issues. For example:
- Data-poverty: A major challenge in soil moisture research is data availability, particularly in tropical regions. Many long-term in situ measurement sites are located in northern latitudes, leaving large data gaps in areas like South America, Central Asia, and Africa. This lack of data makes it difficult to validate satellite-derived estimates and understand soil moisture changes in some of the most climate-sensitive regions.
- Quality of measurements: Some measurements will come from FLUX-NET sites where the focus is on carbon fluxes and soil moisture measurements are often ad-hoc or a by-product.
- Sensor Malfunctions: Long-term records often contain periods where sensors degraded or failed, leading to unreliable readings.
- Calibration Changes: A switch in sensor technology or recalibration can cause sudden shifts in the data, making it hard to trust trends over time.
- Local Biases: Measurements from research sites may not be representative of broader regional conditions.
These factors mean that blindly trusting in situ observations can be misleading. Instead, we must carefully examine time series data to identify inconsistencies before using them for trend analysis.
The Role of Machine Learning in Soil Moisture Analysis
To address these challenges, we are now leveraging machine learning algorithms to merge datasets more effectively. These models align the statistical distributions of different sensors so that no additional bias correction is needed. The result is a more consistent and reliable long-term soil moisture dataset, allowing for better analysis of trends and seasonal variations.
CASM: A long-term Consistent Artificial-intelligence based Soil Moisture dataset on machine learning and remote sensing.
CASM (Consistent AI-based Soil Moisture) is based on the NASA Soil Moisture Active Passive (SMAP) data but is designed to extend the high quality of SMAP soil moisture data back in time, allowing for reliable long-term trend analysis. It isolates anomalies—such as extreme events—by removing the dominant seasonal cycle, significantly improving accuracy. CASM merges multiple satellite datasets using machine learning, leveraging diverse sensor angles and applying transfer learning to maintain SMAP-like quality even before its 2015 launch. It also quantifies uncertainty by accounting for both observational noise and algorithmic error, ensuring robust and reliable soil moisture estimates.
Comparing CASM SSM with other long-term global SSM products
The CASM Soil Moisture dataset demonstrates strong consistency with SMAP SSM, one of the most trusted soil moisture datasets, particularly after 2010 when more advanced L-band sensors became available. Unlike some merged products that show inconsistencies over time, CASM maintains a stable global trend (both temporarily and spatially) and effectively captures variability while minimizing noise from older sensor generations.
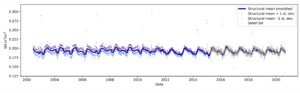
Final Thoughts
Soil moisture is a key variable in understanding climate change, ecosystem dynamics, and agricultural productivity. However, measuring and interpreting soil moisture requires a careful balance between in situ precision and remote sensing coverage.
By leveraging machine learning techniques and carefully merging datasets, we are improving the reliability of long-term soil moisture records. At the same time, acknowledging seasonal variations and addressing data gaps in underrepresented regions will be crucial for advancing our understanding of global soil moisture dynamics.
As research continues, new methods will help refine soil moisture monitoring, ensuring better predictions for agriculture, water resource management, and climate change adaptation.