Announcement of Opportunity: University of Reading Research Programme on Advancing the Frontiers of Earth System Prediction (AFESP) – in partnership with ECMWF, the UK Met Office and NCAS.
This opportunity closed on 26 July 2023. Applicants will be notified of funding decisions in October 2023 and awards publicised by late 2023.
The University of Reading is pleased to offer six five-year research grants to commence late 2023 or early 2024. These grants will deliver research priorities aligned to the strategic science plan for Advancing the Frontiers of Earth System Prediction (AFESP) which has been jointly agreed by the programme partners – the University of Reading, the European Centre for Medium-Range Weather Forecasts, the UK Met Office, and the National Centre for Atmospheric Science.
AFESP Announcement of Opportunity – Important clarification concerning co-development of proposals
With reference to the paragraph below in the AO, we would like to emphasise that all PIs should contact AFESP-Research@reading.ac.uk as soon as possible to enable the co-development of proposals with suitable co-investigators at ECMWF, Met Office and NCAS.
“The AFESP Liaisons Group, chaired by Professor Pier Luigi Vidale, is available for consultation and will assist in identifying co-investigators at ECMWF/MO/NCAS and defining aligned resources. Please contact AFESP-Research@reading.ac.uk ahead of submission (and no later than one month before the call deadline) to be connected with the Liaison Group.”
What we are looking for
Under this Call for Proposals, the programme will support two five-year research projects in each of AFESP’s major themes (six in total):
- Theme 1: Predicting the Earth System up to the sub-seasonal range
- Theme 2: Challenges and opportunities in simulating the Earth System at the kilometre-scale
- Theme 3: Data assimilation for the Earth System across a range of scales
Proposals which seek to address challenges under more than one theme are also welcome. All proposals should also address the cross-cutting theme: Maximising the societal benefits of extended-range numerical weather predictions.
Each project will support:
- A post-doctoral research assistant for five years (A cohort of six PDRAs will be recruited in autumn 2023 with the involvement of the grant-holding PIs; Named researchers will not be accepted)
- Travel and subsistence
- Exceptional costs if clearly justified in the proposal
Each proposal must include:
- A Principal Investigator at the University of Reading (with optional Co-Investigators), and:
- a Co-Investigator from ECMWF
Proposers are encouraged to consider involving additional Co-Investigators from the Met Office and/or NCAS.
NB:
- It is requirement of this call that all collaborating partners contribute significant, in-kind aligned FTE (g. at least 0.1 FTE senior scientist or 0.2 FTE PDRA time).
- All investigators should be involved in a maximum of two proposals and (for UoR staff) named as the Principal Investigator on only one.
Proposals may also include additional Co-Investigators from other institutions where there is significant in-kind aligned resource (as above).
How to apply
Applicants should refer to the Advancing the Frontiers of Earth System Prediction: Strategic Science Plan before preparing their application.
All applicants are required to submit a one-page Expression of Interest by Friday 23 June 2023 to AFESP-Research@reading.ac.uk (For peer review planning purposes and will not be assessed.) Please use the template provided in Appendix 1.
Please submit your full proposal by Wednesday 26 July 2023 directly to AFESP-Research@reading.ac.uk.
Full proposals must be a maximum of eight sides of A4 (Arial, pt11 and 2cm page margins) and include the following:
- Description of the research project. This must be no more than five sides of A4 and include all necessary tables, figures, Gantt chart, and references. The case must highlight the Long-term (15 year) context of the research and make explicit which theme(s), challenges and other aspects of the strategic science plan will be addressed.
- Outline of technical requirements and data management plan. This must be no more than one side of A4 (See separate guidance in Appendix.2)
- Description of the applicants/teams’ skills and capability to deliver. This must be no more than two sides of A4 and should demonstrate the ability of the team to conduct ground-breaking, innovative research and that they have the capability to successfully execute the project and contribute to achieving the long-term ambitions of the AFESP programme.
Additional documents that must be submitted with the proposal:
- Summary of the aligned resource from ECMWF/MO/NCAS/other partners. This must be no more than one side of A4, using the template provided in Appendix 3.
- A Statement of Collaboration and Support from each partner is required to demonstrate aligned partner involvement, named staff & assigned FTE and technical contributions (HPC/data/analysis): This must be no more than one side of A4 per partner institution.
The AFESP Liaisons Group, chaired by Professor Pier Luigi Vidale, is available for consultation and will assist in identifying co-investigators at ECMWF/MO/NCAS and defining aligned resources. Please contact AFESP-Research@reading.ac.uk ahead of submission (and no later than one month before the call deadline) to be connected with the Liaison Group.
Detailed costs are not required. Awards will cover PDRA salary costs (at Grade 6) for the duration of the project and £6,000 per year to cover travel and subsistence. Additional exceptional costs may be considered on a case-by-case basis and applicants should discuss these with the AFESP Programme Manager ahead of submission (c.wyllie@reading.ac.uk).¹
Projects are expected to start no later than 1 February 2024.
How we will assess your application
We will assess your proposal using a two-stage panel-based independent peer review process.
- Stage one: Expert written review and short-listing
Proposals will be assigned to Peer Review Panel members to prepare written reviews with appropriate scoring (2 or 3 per proposal). External reviews will be sought if additional expertise is necessary.
Depending on the number of applications received, the Panel may meet to decide which applications to invite to interview stage. All applicants will receive written reviews but will not be required to respond in writing. Instead, those applicants invited to the interview will be asked to address review comments in their presentations.
- Stage two: Panel interviews (one- or two-day panel meeting)
Shortlisted proposals will be invited to a 30-minute interview (10-minute presentation plus 20-minute Q&A) with the Peer Review Panel. The panel will assess and rank proposals and advise the Executive Management Group on which proposals should be funded. Final decisions lie with the Executive Management Group.
Criteria for assessment
Research excellence and risk/reward
- Does the proposal present a compelling case for research that is ambitious, timely and will advance the frontiers of knowledge over a time-scale compatible with the strategic nature of AFESP?
- Are the outlined scientific approaches feasible and the high-risk aspects well considered and managed?
Fit to programme
We will assess whether the proposal:
- Clearly highlights the long-term and international context of the research.
- Makes explicit which theme(s), challenges and other aspects of the AFESP strategic science plan will be addressed and how.
- Demonstrates how it will contribute to development of the wider research field.
- Involves well-considered collaborations with partner organisations (ECMWF/MO/NCAS/etc.)
Proposals that do not demonstrate a strong fit will not be funded.
Capability to deliver
To what extent:
- Does the team demonstrate appropriate expertise and capability to successfully execute the proposed project and contribute to addressing the long-term ambitions of AFESP, including evidence for capacity to support and mentor researchers involved as appropriate.
Value For money
- Are there significant aligned resources from partner organisations (ECMWF/MO/NCAS/etc.) and are these well described and quantified?
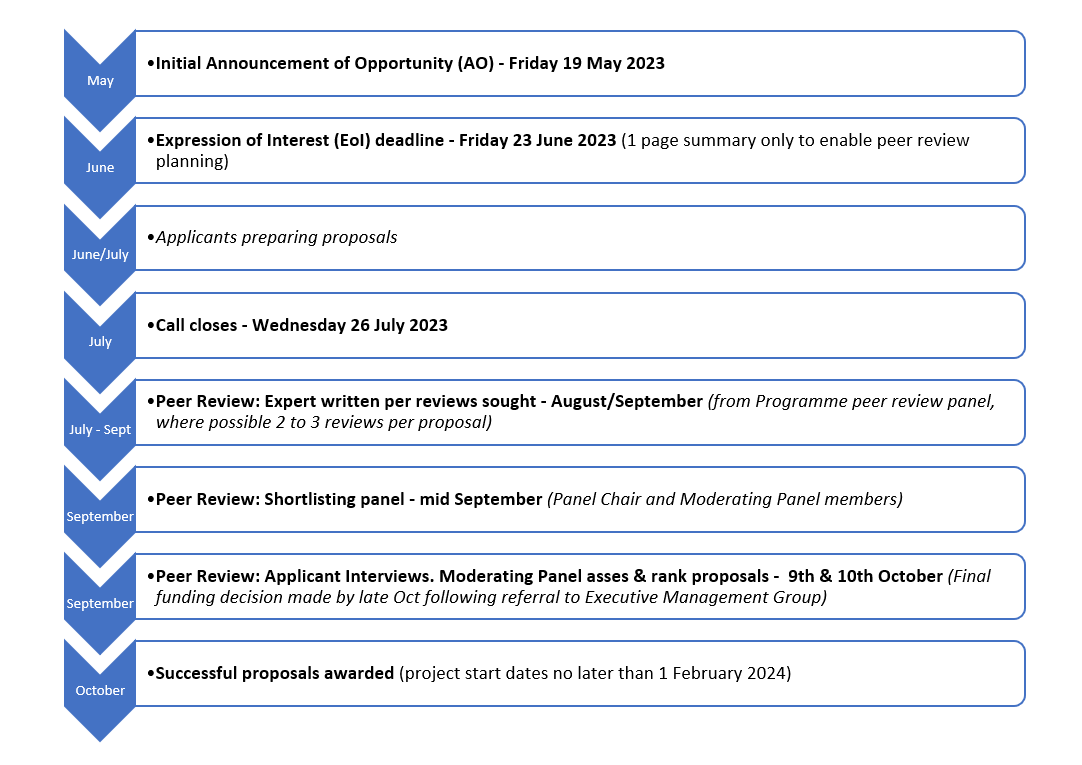
¹ For UoR academic staff Principal Investigator/Co-Investigator time is not directly funded but there is the possibility that this can be included in workload allocations with agreement from relevant Heads of Department.
For externally funded permanent UoR staff it may be the case that acting as an AFESP Investigator is compatible with the terms and conditions of their external funding (i.e as an in kind contribution) and relevant staff are encouraged to explore this possibility. However, if this is not possible then externally funded UoR Investigators can include 0.1 FTE as an exceptional cost. This will require a clear statement that undertaking this role will not conflict with obligations to the external funder, including a justification of how the externally funded work will be impacted (e.g. will a project duration be extended, or will another member of staff undertake some part of it.) If there is more than one externally funded UoR Investigator then it will be important that the associated FTE is carefully justified.