Language models like ChatGPT force us to consider what we are prepared to treat as meaningful language users. For World Philosophy Day 2023, Jumbly Grindrod explores the importance of the philosophy of language and how contemporary developments can breathe new life into older debates.
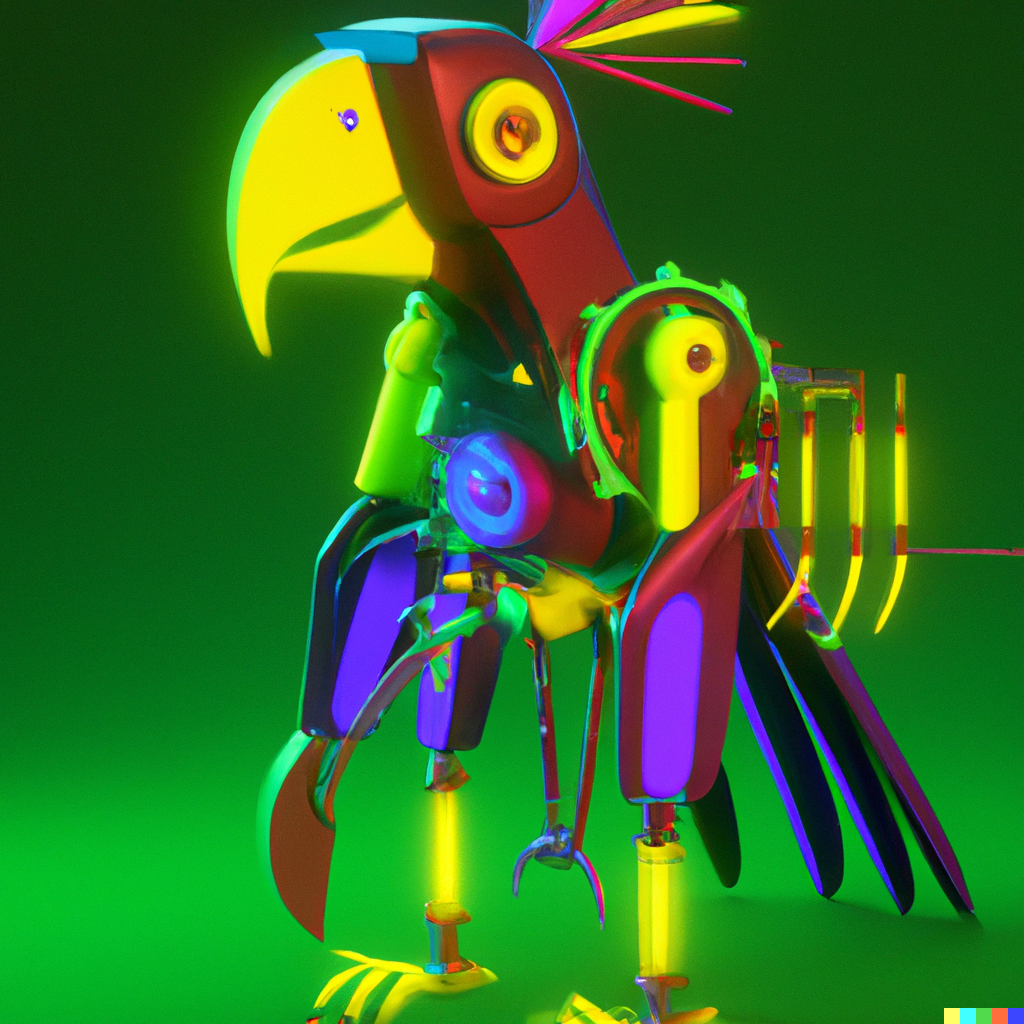
One of the great things about philosophy is that you have the opportunity to explore a vast intellectual history, stretching back to ancient times, while also trying to make sense of contemporary problems. Indeed, sometimes old questions return in new guises, breathing life into debates that seemed at a historical standstill. As a philosopher of language, I want to show just one example of this in my research which focuses on large language models like ChatGPT.
Anyone who has had the opportunity to converse with ChatGPT will be struck by its sophistication. And it is tempting to think that ChatGPT must be different to previous computational systems in that it can actually understand a language and use words meaningfully. In popular discussion on the topic, there has been a tendency to conflate the following questions:
- Is ChatGPT conscious?
- Is ChatGPT an instance of general artificial intelligence?
- Can ChatGPT believe or intend anything?
- Does ChatGPT understand what it writes?
- Can ChatGPT use words meaningfully?
In philosophy we treat 1-5 as distinct: an answer to one may not lead to an answer to the others. I have largely assumed that the answers to 1-4 will be negative: when you look at how language models are developed, they just don’t look like systems that are capable of having mental lives.
How large language models work
Language models are trained on huge bodies of text. This text is completely unannotated – meaning there is no extra information accompanying it. Think of a .txt file that any of us could open on our laptops. Using this text, the model trains itself on a kind of missing word task, where it covers up a word in a sentence, and then predicts what the missing word is. It can do this because for each word, it constructs an increasingly sophisticated account of the kinds of words that it turns up next to.
So, at the core of large language models is a system that is sensitive to how words go together, and it uses this information to mimic human language use by generating statistically-plausible text. The great question about the progress in language model technology is really how far this kind of approach can take you.
What is a meaningful language user?
Nevertheless, I have been exploring whether language models could still be considered as meaningful language users. Some researchers are sceptical about this. Emily Bender and Alexander Koller, for instance, view language models as stochastic parrots. Just as a parrot who is able to chirp “Oh there is blessing in this gentle breeze” does not really use the words in any meaningful sense, they argue that language models are merely mimicking proper language use.
But here are two (mutually reinforcing) reasons why the issue is a bit more complicated than that. The first reason is empirical. There has a been a great deal of work in computational linguistics to investigate what kind of information has been stored in large language models (for example, see here and here). And those findings suggest that in fact a great deal of linguistic information has been stored, even though this information was not explicitly represented in the original training data. This includes part-of-speech categories (whether an expression is a noun, verb, determiner etc.), (the grammatical relations held between expressions within a larger phrase or sentence), (for example, whether an expression is a patient or agent of some verb phrase) and co-reference (whether two expressions refer to the same object). So language models infer information that is only implicit within its training data, where this stretches to quite sophisticated linguistic properties. The parrot has obviously not done this.
The second reason is more philosophical. There is a widely-held view in philosophy of language that whether a speaker meaningfully uses a word doesn’t just depend on their internal psychology but also on the external environment that the speaker is in. The view is called externalism. There are many ingenious arguments for externalism, but we can start to see the strength of the view by considering whether ordinary speakers always have perfect grasps of the words that they use.
For instance, I don’t know what the essential feature of the chemical element molybdenum is (presumably some chemical weight), but I am still able to use “molybdenum” to talk about the same stuff that chemists talk about in a way that the parrot cannot. One plausible suggestion is that facts about the linguistic community I belong to (and that the parrot does not) determine that I can (and the parrot cannot) use the word to talk about the stuff.
Maybe language models use words in the same way that I use words like “molybdenum”. It doesn’t have perfect access to the defining features of the word, but it does engage with the words in the right way to count as a meaningful writer. To see whether this is true, we need to investigate the notion of a proper language user, and this is partly a philosophical investigation with a history that stretches back through some classical debates in philosophy of language.
Jumbly Grindrod is Lecturer in Philosophy at the University of Reading.