DARE
Data Assimilation for the REsilient City (DARE) was a research project and network funded by an EPSRC Senior Fellowship in Digital Technology for Living with Environmental Change. The project has now ended and this website is no longer being updated.
Data assimilation is an emerging mathematical technique for improving predictions from large and complex forecasting models, by combining uncertain model predictions with a diverse set of observational data in a dynamic feedback loop. This video tells you more about the project legacy. For more information about the research programme click here.
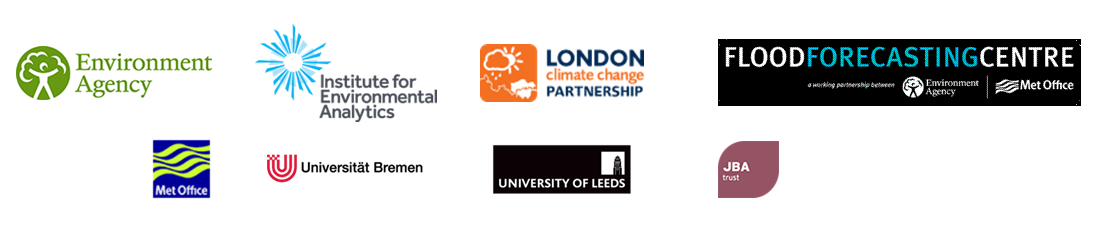