Takuya Kurihana has received funding from the DARE training fund to attend Data Assimilation tutorials at the Workshop on Sensitivity Analysis and Data Assimilation in Meteorology and Oceanography, 1-6 July 2018, Aveiro, Portugal. Here he writes about himself and his research.
What if we could more accurately predict what atmospheric phenomenon will happen in the next minute, hour and day using the current limited information. This scientific question has inspired me to be being involved in the research activity since undergraduate student. I am Takuya Kurihana, a Meteorology MS student in the University of Tsukuba under the supervision of Dr. Hiroshi L. Tanaka, and an incoming Computer Science PhD student in the University of Chicago. My current research focuses on 1. How to improve the accuracy of weather forecasting: “Predictability”, and 2. How to make use of a massive amount of dense meteorological dataset for data assimilation. With developing new application for purpose 2, I am now researching the impact of using a large amount of atmospheric observation as much as we can towards the daily scale weather forecast.
Regarding to the improvement predictability, as a previous article explained by Zak Bell, Making the Most of Uncertain Urban Observations , data assimilation plays an imperative role in numerical weather prediction because the longer we run a numerical weather forecasting model, the larger the error of forecast grows up. This is because the uncertainty. Even if we use the most precise model, this tendency would not change more or less. But, applying the data assimilation methods can minimize the error by installing observation into the optimization process Fig.1 is an example experiment about an advantage of data assimilation. Therefore, we have to gather a variety of denser observation data from both horizontally and vertically wider range of points in real operation. Other than land observation (Figure 2) [1], sondes, and buoys, recent satellite observation (Figure 3 and Figure 4) [2, 3], which provide us much richer and denser information, have been utilized in operational data assimilation processes.
The spatially condensed satellite data, however, causes one problem in the current data assimilation methods. The issue is that too much dense data will rather deteriorate the quality of assimilation products based on previous researches. Simply put, we have to leave out large proportion of these data: “Thinning”, even while the technology of meteorological satellites is advancing. Moreover, there are several resource limitations to prepare the forecasting since we are not afford to compute endlessly, and the performance and size of computer are constrained. In order to make use of larger proportion of these data while not reducing assimilation quality, the spatial interpolation, so called super-observation (SO) procedure are developed. As one SO system, I proposed a new algorithm which could deal with a massive amount of satellite big data efficiently and speedily within a cloud-resolving model (Nonhydrostatic ICosahedral Atmospheric Model; NICAM) grid coordination. The algorithm primary targets to reduce “Do/For Loop” iteration process to find the nearest model grid location, which can also skip the computation by a complex observation operator.
Which is better Thinning or SO? Although this would be controversial discussion among meteorologists, I would like to give one example in the Workshop on Sensitivity Analysis and Data Assimilation in Portugal. While the new application should be tested in further numerical experiments through my master research project, I ponder that we should consider a more efficient usage of these meteorological “Big Data” in the near future. Through the attendance at the workshop, I would like to discuss my application and its effect on the data assimilation, as well as receive fruitful advice from cutting edge researchers.
Figure 1. These timeseries of trajectories imply a small difference between two initial conditions finally ends up completely varied behaviors. Blue is No data assimilation from 200 time steps, and Red is data assimilation Lorenz63 Trajectory. Demo above by Takuya Kurihana.
Figure. 2 This map shows the sparse location of land observation points
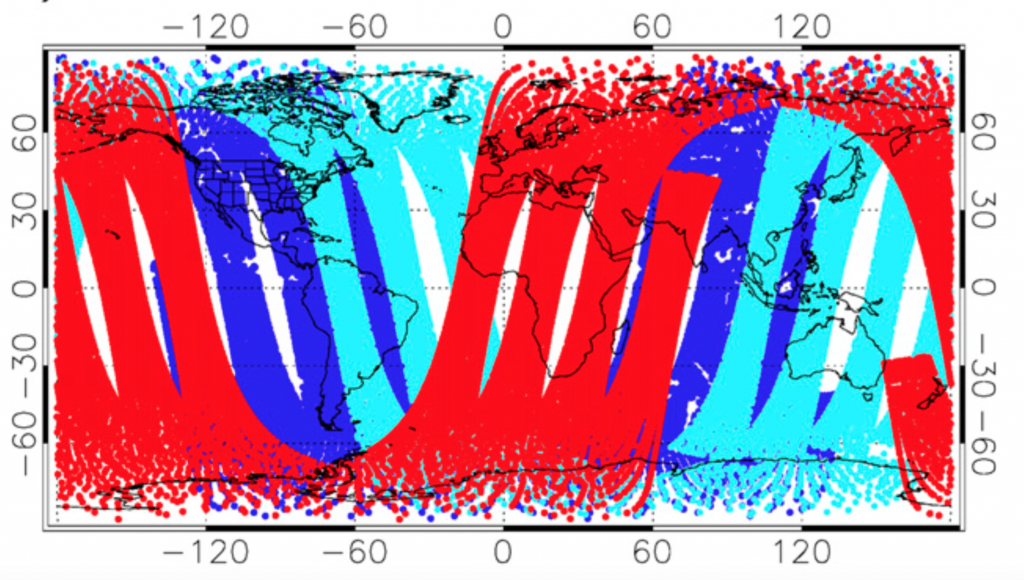
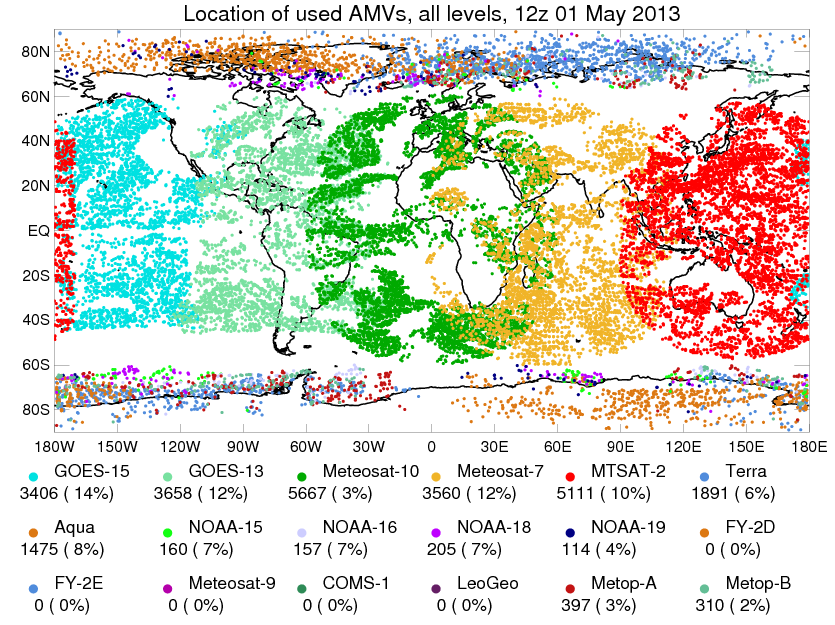
[2] S-A. Boukabara, K. Garrett, K. V. Kumar, “Potential Gaps in the Satellite Observing System Coverage: Assessment of Impact on NOAA’s Numerical Weather Prediction Overall Skills”. (2016). Mon. Wea. Rev., 144, 2547–2563, https://doi.org/10.1175/ MWR-D-16-0013.1.
[3] http://www.eumetrain.org/data/4/438/navmenu.php?tab=2&page=2.0.0