by Gabriella Giannachi, Steve Benford and Lydia Farina
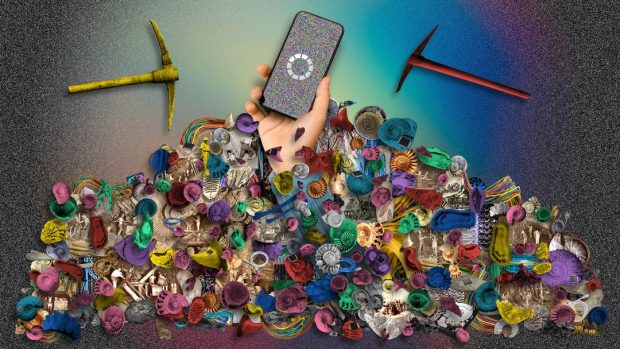
Archives have changed throughout history often in response to the introduction of novel technologies. At the heart of the BRAID/AHRC-funded project ‘Creating a Dynamic Archive of Responsible Ecosystems in the Context of Creative AI’ (2023-4), has been the question as to what might archives become in the future when AI will most probably form part of several artworks that will be archived by different kinds of cultural organisations which will also most likely use AI for the documentation and conservation of such artworks. The project, led by the University of Nottingham in collaboration with the University of Exeter, National Archives, and Blueskeye AI, investigated how archives could become dynamic so as to accommodate these kinds of artworks, and related documentation and archiving practices, looking also into what ecosystems are necessary for the production and conservation of this kind of archive.
Building on the curator and new media researcher Annet Dekker’s findings about how best to conserve artworks by using a ‘network of care’ (2018) and Dekker and Giannachi’s notion of ‘generative preservation’ as a way to enable further iterations of existing artworks as part of the conservation process (2023), we started by looking into who might be the possible stakeholders of such archives, mindful of the fact that the ecosystem of an artwork and the ecosystem of its documentation and archive may differ depending on whether the artwork is preserved by the artists, a library, a museum, or all of the above. This suggests that the key stakeholders of a work will be its creators, performers, funders, copyright-holders, and end-users, as well as the stakeholders from the archives and collections in which the works may reside.
Conscious of AI’s potential to hallucinate especially when faced with incomplete and recent data, we arrived at the conclusion that dynamic archives should be editable and facilitate annotation; continue to grow over time; reconstitute themselves in accordance to keywords set by whoever may wish to consult them; make visible different time-based versions of themselves; be used curatorially and creatively as a live (generative) archive; and provide the context and live, iterative, documentation of a work, including artworks generated by AI. Interestingly, when we asked ChatGPT what it thought a dynamic archive was, it described it as an evolving and interactive repository of different types of resources facilitating personalisation (or customization), agility, contextualisation, collaboration and feedback — a definition that was not too far from the one we had arrived at. Critical, is the fact that these archives’ ecosystems should make room for unpredictable factors that might emerge over time. In this sense, ecosystems must remain open and be capable of anticipating change.
We found that the potential of AI in documentation is related to what has already been documented and is available to it. This means that the use of AI for contextualisation, audience documentation, the documentation of a large quantity of data pertaining to different iterations of a work and the documentation of change, which are key parameters that current documentation practices struggle with, as well as the use of AI in the generation of ‘live’ dynamic archives, could all be built on, provided the AI has access to these documents. Moreover, AI could process documentation specifically in relation to different stakeholders paying attention to the individual actors and groups interacting with each other and with the AI both at a specific moment in time and iteratively. This means AI can be used to produce generative archives which are placed both within the fields of conservation and the creative industries.
In conclusion, we found that AI can generate documents, artworks, further iterations of those works, and their archives, and keep them connected among each other, operating as a time machine. This shows that AI could be extremely useful for the documentation, generation, and preservation of intangible cultural heritage, but only as far as it is cared for by healthy and deep ecosystems. In this, we must ensure that AI as a creator, producer, generator and documenter of a work showcases the priorities of responsible innovation (Jirotka et al. 2017). Hence, the design process of a dynamic archive should be inclusive of different stakeholders and should anticipate risks and challenges associated with the use of huge volumes of other incomplete data. This means that a healthy and deep AI ecosystem, should be invested not only in a trustworthy but also an ethical, accessible, sustainable, and EDI-oriented AI so that it could itself, as a human-AI entanglement, promote EDI while remaining mindful of ethics, accessibility, and sustainability.
Acknowledgments
We gratefully acknowledge BRAID/AHRC for funding ‘Creating a Dynamic Archive of Responsible Ecosystems in the Context of Creative AI’.
Works cited
Dekker, A. (2018) Collecting and Conserving Net Art. Moving Beyond Conventional Methods. London: Routledge.
Dekker, A., and Giannachi, G. (eds) (2023) Documentation as Art, London and New York: Routledge.
Jirotka, M., Grimpe, B., Stahl, B., Eden, G. and Hartswood, M. (2017) ‘Responsible research and innovation in the digital age’. Communications of the ACM 60, 5 (May 2017), 62–68. https://doi.org/10.1145/3064940