By Melanie Ades
The Copernicus Atmosphere Monitoring Service
The Copernicus Atmosphere Monitoring Service (CAMS) is one of six data services under the Copernicus Earth Observation (EO) programme coordinated by the European Commission. The aim of the services is to transform the wealth of satellite EO data into timely and actionable information by processing and analysing it. The specific focus of CAMS is global air pollution and health, solar energy, greenhouse gases and climate forcing.
EO data are often complex and do not directly measure the quantity of interest. For example, one of the key measures of air quality used by governments to set targets for cleaner air is fine particulate matter (PM2.5) at the Earth’s surface. What is measured by satellites is the extinction of the suns light through a column of the full atmosphere because of particles, such as dust and haze. CAMS uses data assimilation to transform satellite observations into consistent and quality-controlled information on the specific values that are needed by policymakers, businesses and research organisations.
Data assimilation in the global service
The global CAMS service is managed by European Centre for Medium-range Weather Forecasts. It uses the Integrated Forecasting System (IFS) to model both the weather and atmospheric composition. The data assimilation method used as part of the IFS for numerical weather prediction (NWP) is incremental 4DVAR (Courtier et al., 1994). This method is extended to atmospheric composition by adding key observed chemical species to the variables being optimised (Flemming et al., 2017). However, what makes data assimilation for atmospheric composition interesting is not how it is done but the challenges that add complexity compared to NWP.
1. Few chemical species observed
One of the first challenges is that there are over one hundred different chemical species modelled as part of CAMS, of which only six are observed. There are so many different chemical reactions between these species in the full atmospheric composition model that they cannot all be represented in the data assimilation process.
In fact, in CAMS currently only one vital process is included, which is a simple representation of the conversion of nitrogen dioxide to nitrogen oxide in daytime. Although this one reaction is necessary to be able to use the satellite observations of nitrogen dioxide, balancing the benefit of including more of the chemical reactions versus the computing time required for operational forecasting is still an area of active research.
2. Mismatch between observations and model equivalents
There is also a mismatch in atmospheric composition between what is observed by the satellite instruments and what is modelled on the computer. This is also true to a certain extent in NWP but is more pronounced in atmospheric composition.
One example is that most observations received from satellites for atmospheric composition are total column observations, which means they have little information on how a chemical is distributed through the atmosphere. Therefore, the uncertainty in the modelled profile of the chemical becomes very important in the distribution of the observational information. If the uncertainty is not represented accurately then data assimilation increments will be produced at the wrong height and will not improve the forecasts.
Another example is the measurement of the extinction of the suns light because of small particles or aerosols in the atmosphere (Aerosol Optical Depth (AOD)), such as dust and ash. The model separates out dust and ash because they are generated through two quite different processes. Smoke depends on the emissions from fire and dust on the wind at the surface. However, the satellite just observes that the full power of the sunlight is not being reflected off the surface of the earth and knows nothing about which type of particle may be obscuring the suns light. This information must come from the model and can lead to data assimilation increments in the wrong aerosol species when the model does not accurately represent reality.
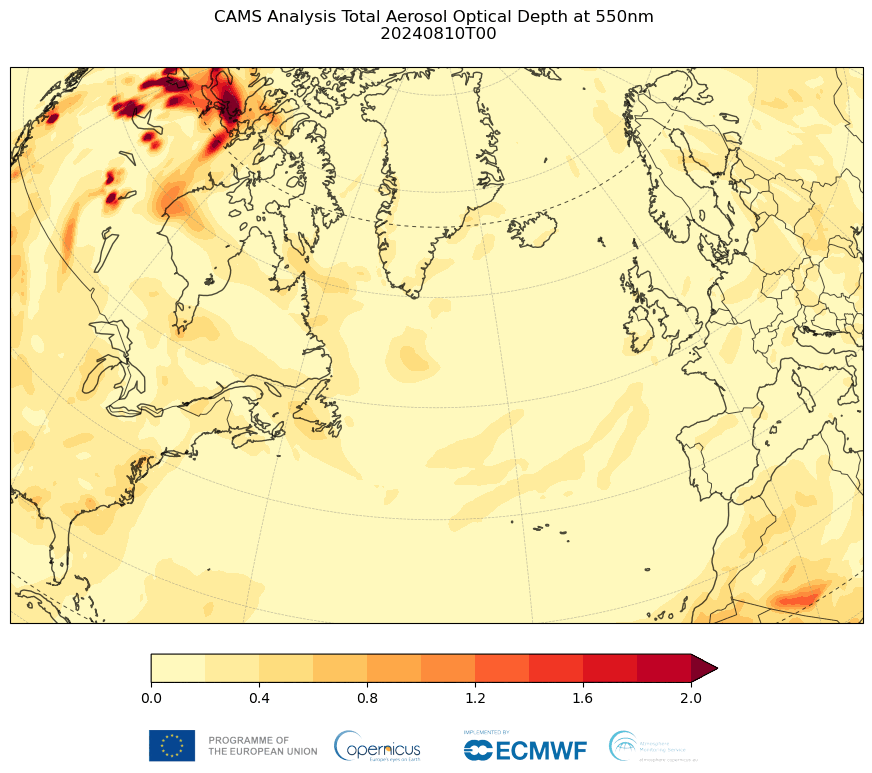
3. Emissions as an external forcing
Finally, emissions play a hugely important role in atmospheric composition. In NWP the aim is to find the initial value for the model variables that most closely matches all the available data. In atmospheric composition the emissions can be as important as the initial value. Taking again the example of smoke from a forest fire, CAMS looks at observations in a 12-hour period to update its initial condition, but the amount of smoke emitted by a forest fire may change a lot in 12 hours and it is never going to be possible to capture this just by changing the initial conditions.
Similarly, one of the primary areas covered by CAMS is greenhouse gases and climate forcing. A new carbon dioxide Monitoring and Verification Support (CO2MVS) service has been launched under CAMS that will build on the current data assimilation system to include emissions. The aim is to provide consistent and reliable information to help monitor greenhouse gas emissions by using satellite observations of methane and carbon dioxide to update emissions through data assimilation.