By Visweshwaran Ramesh
Joint State-Parameter Assimilation
We developed a joint state-parameter assimilation framework by simultaneously updating both the Pedotransfer Function (PTF) constants (which link to JULES’s hydraulic parameters) and the initial soil moisture conditions. Pinnington et al. (2020) previously developed the Land Variational Ensemble Data Assimilation Framework (LAVENDAR) for parameter estimation in JULES, which we modified to update both the state and parameters. This integrated approach manages uncertainty more effectively and represents the first application of this method in JULES, with the goal of improving predictive accuracy across various soil types in the UK.
Data Assimilation Approach
We adopted the hybrid 4D-Ensemble Variational (4DEnVar) approach, as it enhances land surface applications by capturing non-linear error evolution over time, leading to more accurate state and parameter estimates. It also removes reliance on complex adjoint models and offers greater computational efficiency by integrating ensemble forecasts within a variational framework.
Comparative Study
To demonstrate the advantage of state-parameter assimilation, we compare it with two additional assimilation scenarios: optimizing initial soil moisture conditions (state-only assimilation) and adjusting soil hydraulic parameters by optimizing PTF constants (parameter-only assimilation).
The study examined 16 COSMOS-UK (Cosmic-ray Soil Moisture Monitoring Network) sites across the UK, covering three primary soil types: typical mineral soil, mineral soil with high organic content, and calcareous mineral soil (Figure 1C).
Why COSMOS-UK Data Makes a Difference
Most traditional models, including JULES, rely on pre-set values for soil characteristics that don’t always match real-world conditions. To improve accuracy, we used soil moisture observations from the COSMOS-UK, which provides localized, field-scale measurements across diverse UK landscapes. COSMOS-UK stands out for its ability to capture soil moisture over large areas (up to 25-30 hectares) and at greater depths (up to 80 cm), providing continuous, real-time data (hourly temporal scale) that helps track variations with high accuracy (Cooper et al. 2021).
Improved Accuracy Across Varied Soil Types
The joint state-parameter assimilation brought the best results, significantly improving the model’s accuracy. The Kling Gupta Efficiency (KGE), a standard measure of model performance, rose by 143%, from 0.33 to 0.72 on average across different soil characteristics (Figure 1B). This improvement was particularly pronounced in areas with challenging soil types, such as high-organic soils. Models often struggle with these areas due to complex soil properties, but our approach achieved major accuracy gains, with a maximum improvement of 350% at the GISBN site (Figure 1A).
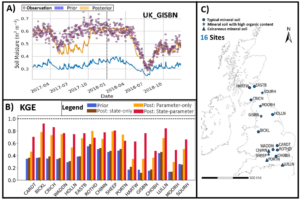
This study marks the first use of joint state-parameter assimilation with JULES, opening new doors for soil moisture prediction in various landscapes. With COSMOS-UK data enhancing its precision, the model is better equipped to support sustainable land management and inform decisions in water resource planning, flood control, and agriculture.
References
Pinnington, E., et al. (2020). The Land Variational Ensemble Data Assimilation Framework: LAVENDAR v1.0.0, Geoscientific Model Development, 13, 55–69. https://doi.org/10.5194/gmd-13-55-2020
Cooper, E., et al. (2021). Using data assimilation to optimize pedotransfer functions using field-scale in situ soil moisture observations, Hydrology and Earth System Sciences, 25, 2445–2458. https://doi.org/10.5194/hess-25-2445-2021