by Tim Trent
When thinking of topics for this blog, I decided to take a reflective approach. Just reviewing past blog entries, the levels of effort and complexity needed in looking for uncertainty closure are apparent to the reader. Furthermore, the project makes a clear case for the importance of why we should care about the uncertainty of the measurements we use. As we enter the last phase of the project, focus is switching towards how to apply the FIDUCEO metrological concepts to satellite climate data records. This topic is of great significance to international efforts, such as the European Space Agency (ESA) Climate Change Initiative (CCI), that aims to provide quality datasets which realise the potential of global long-term Earth Observation (EO) archives.
With this in mind, this raises the question “What will the legacy of FIDUCEO be?” This question will have different answers for different communities and thus is maybe not quite so straight forward. However, perhaps the truth is that it will and should be multifaceted. From my perspective, the common thread that runs through all possibilities is the fully described uncertainty budget estimate. So why do I think this? Whether we study the carbon, nitrogen, water, energy, or any of the environmental cycles, closure of these budgets allows us to improve our understanding of the Earth system. With NASA Decadal survey focusing on process-oriented studies, fully capturing the uncertainty from EO will let us test our knowledge at each step on a global scale.
Uncertainty closure assessment is now becoming a key component in validation studies [1]. Within Water Vapour CCI (WV_cci) total column water vapour (TCWV) products are being assessed against GPS ground-based receivers as part of the retrieval performance assessment. This match-up exercise not only uses the uncertainty from the in situ (m_{2}, u_{2)} and satellite products ( m_{1}, u_{1} ) ; it also includes estimates of the representativeness or collocation uncertainty (\sigma ) in the collocation description. Therefore, in line with the Immler formula [2] the system is described thus:
| m_{1} – m_{2} | < k \sqrt{(\sigma^{2} + u_{1}^{2} + u_{2}^{2})}
( k being the coverage factor, which determines an interval about the mean value as a multiple of the standard uncertainty).
Starting from this point, we can begin to visualise this relationship in such a way that we can determine how well we capture the total uncertainty in the system. The first assumption is that, if fully characterised, then k in the Immler equation is equal to 1. Therefore, this assumption would lead to a 1:1 growth in the standard deviation in the observed differences (| m_{1} - m_{2} |) vs the total uncertainty (\sqrt{(\sigma^{2} + u_{1}^{2} + u_{2}^{2})}) Figure 1 demonstrates this concept for satellite TCWV collected over a SuomiNet GPS site in South America from January 2007 to December 2016.

Figure 1: Example of uncertainty validation based on Immler equation [2]. The black dashed 1:1 line (k=1) is constant below 1 kg/m2 as this region is limited by the accuracy of the in situ measurement.
The red bands in the top of the figure show the (+/-) standard deviation as a function of the total uncertainty with the bin densities show in the histogram below. The results at this station look convincing such that we can look at how non-representative collocations affect the scatter in the data with some degree of confidence.
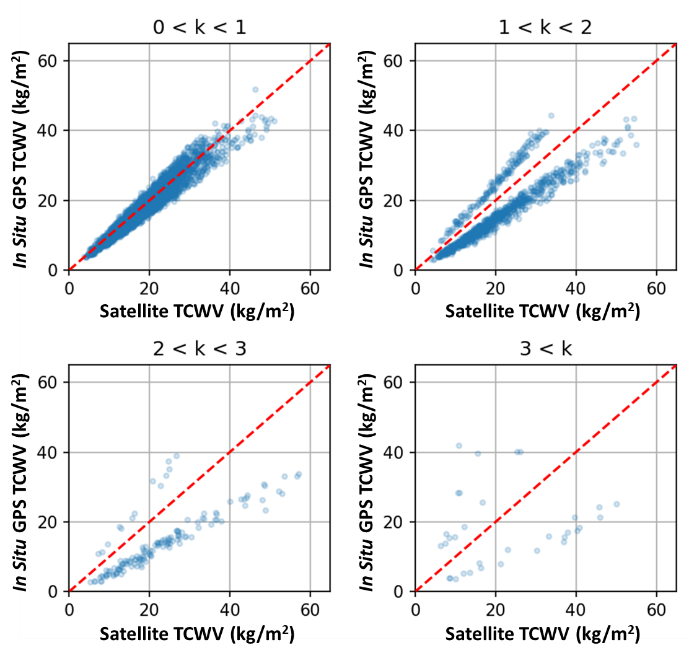
Figure 2: Impact on scatter due to the non-representativeness of outlying collocated pairs. If we have confidence in our uncertainty budget then we can use this criteria to filter data from our analyses.
Figure 2 shows four panels that are a function of k interval. This example demonstrates how the higher the k-value is, the more divergent from the 1:1 (red dashed) line cases become. Now while this may seem intuitive, it allows us to examine the breadth of scattering in the match-ups. Thinking of this an iterative methodology, we can look at/target individual uncertainty components where knowledge gaps present themselves.
It is in this undertaking, where we are looking for closure, that FIDUCEO’s legacy, the tools and resources of the project will serve us well.
References:
[1] Ghent, D.; Veal, K.; Trent, T.; Dodd, E. and Remedios, J., A new approach to defining uncertainties for MODIS land surface temperature. Remote Sensing, 2019, under review.
[2] Immler, F. J.; Dykema, J.; Gardiner, T.; Whiteman, D. N.; Thorne, P. W. and Vömel, H., Reference Quality Upper-Air Measurements: guidance for developing GRUAN data products. Atmospheric Measurement Techniques, 2010, 3, 1217–1231, doi:10.5194/amt-3-1217-2010